LiDAR Measurements
Lidar measurements are essential for validating and improving analytical wake models used in wind energy. These models predict how the wakes generated by wind turbines behave, which is crucial for optimizing wind farm performance. Here’s a detailed guide on how to use Lidar measurements for this purpose:
1. Understanding Analytical Wake Models
Analytical wake models are mathematical representations that predict the velocity deficit and turbulence intensity in the wake of a wind turbine. Common models include:
- Jensen (Park) Model: Simplifies wake expansion and velocity deficit using a linear relationship.
- Larsen Model: Considers turbulence intensity and provides a more detailed wake profile.
- Gaussian Model: Uses a Gaussian distribution to describe the wake velocity deficit.
2. Setting Up Lidar Measurements
Types of Lidar Systems
- Ground-based Lidar: Positioned on the ground to measure wind profiles at various heights.
- Nacelle-based Lidar: Mounted on the turbine nacelle to measure approaching and downstream wind conditions.
- Floating Lidar: Deployed offshore on buoys to gather wind data over the ocean.
Measurement Campaign
- Duration: Conduct long-term measurements to capture varying atmospheric conditions.
- Coverage: Ensure measurements cover the near wake (immediately behind the turbine) and the far wake (further downwind).
3. Data Collection
Parameters to Measure
- Wind Speed and Direction: At multiple heights and distances from the turbine.
- Turbulence Intensity: To understand the mixing and dissipation of the wake.
- Atmospheric Conditions: Temperature, pressure, and humidity to classify atmospheric stability.
4. Validation of Analytical Wake Models
Data Processing
- Time-Averaging: Average Lidar data over suitable time intervals to reduce noise.
- Spatial Averaging: Aggregate data from multiple measurement points to represent wake characteristics.
Model Comparison
- Velocity Deficit Profiles: Compare measured wind speed reductions with model predictions.
- Wake Width and Recovery: Assess how well the models predict wake expansion and velocity recovery downwind.
- Turbulence Intensity: Validate model predictions against measured turbulence levels.
Statistical Analysis
- Error Metrics: Use metrics like Root Mean Square Error (RMSE) and Mean Absolute Error (MAE) to quantify model accuracy.
- Correlation Analysis: Assess the correlation between measured and predicted wake characteristics.
5. Improvement of Analytical Wake Models
Model Calibration
- Parameter Adjustment: Modify model parameters (e.g., wake decay constant, turbulence intensity factors) to improve fit with Lidar data.
- Atmospheric Stability Incorporation: Include terms or factors that account for stable, neutral, and unstable atmospheric conditions.
Enhanced Model Development
- Hybrid Models: Combine elements of different analytical models to capture various wake effects more accurately.
- Data-Driven Models: Incorporate machine learning techniques using Lidar data to develop more accurate predictive models.
6. Practical Application
Wind Farm Design Optimization
- Turbine Placement: Use improved models to optimize turbine spacing, reducing wake losses.
- Yaw Control Strategies: Develop adaptive control strategies to mitigate wake impacts on downstream turbines.
Operational Adjustments
- Real-Time Monitoring: Implement systems that use Lidar data for real-time wake monitoring and turbine control adjustments.
- Maintenance Planning: Predict and address increased wear and tear on downstream turbines due to wake effects.
Conclusion
Using Lidar measurements to validate and improve analytical wake models significantly enhances the accuracy and reliability of these models. This leads to better wind farm design, optimized turbine operation, and ultimately, increased energy production and efficiency. Continuous advancement in Lidar technology and data analytics will further refine wake modeling, supporting the growth and sustainability of wind energy.
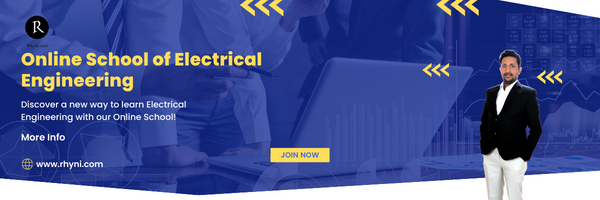